
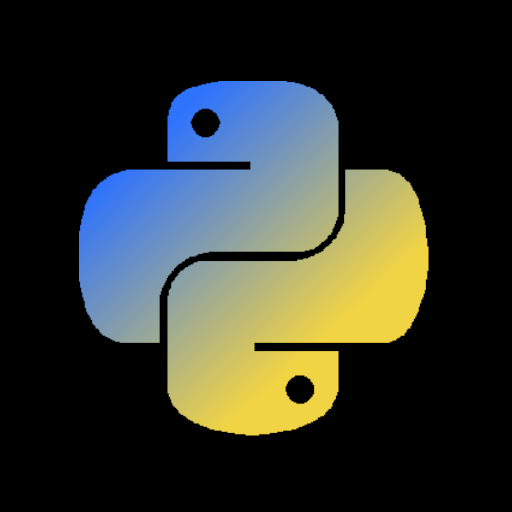
You don’t have to convince me that Rust rocks. I just need to convince my team that it’s worth the investment in terms of time to onboard everyone, time to port out application, and risk of introducing bugs.
We have a complex mix of CRUD, math-heavy algorithms, and data transformation logic. Fortunately, each of those are largely broken up into microservices, so they can be replaced as needed. If we decide to port, we can at least do it a little at a time.
The real question is, does the team want to maintain a Python or Rust app, and since almost nobody on the team has professional experience with low-level languages and our load is pretty small (a few thousand users; b2b), Python is preferred.
And yet, if someone asks, I will link it. I’m not proud of it, but I am helpful to a fault.