
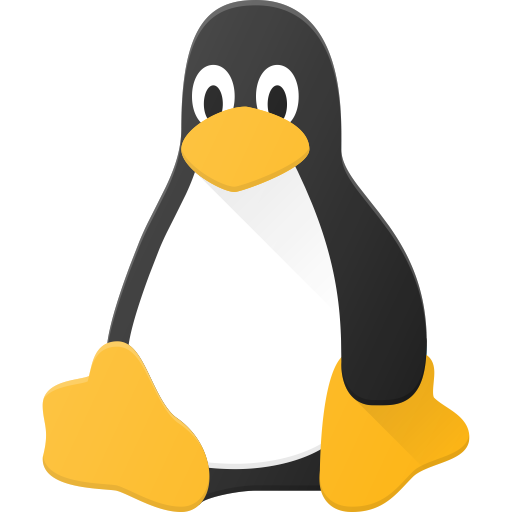
All stdout gets piped through lolcat now.
pointless
All stdout gets piped through lolcat now.
It might be due to display drivers not playing nice on wayland; I’ve had this problem a couple months ago on arch with the amdgpu drivers, using either one of sway, labwc, or KDE. It did not happen on Xfce, though; and after a kernel update the problem subsided.
I was about to say the same – and also: nftables syntax is a lot cleaner compared to iptables, and the whole configuration can be loaded from a single file just like pf, without doing the dump/reload cycle that iptables required. Unless UFW does features like defining zones which a user might need (like firewalld), then it’s not a huge improvement on bare nftables usability-wise.
This is the right response to the OP’s bizarre “question”, of course, but … yeah … the ‘for the most part’ qualifier is key here.
Some caveats, though: To share the same home folder safely, it’s best to use the same desktop environment on both distros. Debian paired with Fedora makes it difficult to match the release numbers of the desktops, though, and there might be discrepancies with respect to user config files in the home folder, when you’re trying to configure features in Fedora that aren’t yet available in Debian.
Also the system folder setup (locations of libraries and include files) is different between the two, so if there’s anything in the home folder that’s linked against libraries in one distro, it won’t work in the other. Especially if you’re going to compile anything in the home folder – including stuff that package managers of scripting languages like lua and python themselves compile – that could lead to major heaadaches.
I don’t think it does virtual desktops with labwc still; but when it does, labwc is as good a replacement for xfwm as any, IMHO.
labwc can do virtual desktops; there’s a desktop switcher, and the window switcher is aware of windows only in the current desktop – but I can’t figure out how to query window-per-desktop information programmatically otherwise. waybar, wlrctl, as well as xfce-panel don’t seem to have access to that info either. Still waiting for accomodations with respect to some wayland extension, I suppose.
Ubuntu’s font rendering used to be better than every other distro, because they incorporated patches on freetype that were legally ‘iffy’ as to whether they infringed on microsoft’s patents; later whatever exclusivity requirement that there was with those patents expired, and the patches got upstreamed in freetype itself.
So now all Linux desktops are capable of subpixel font rendering, hinting, whatever. But before that, font rendering really was hideous on other distros.
Smaller browsers built on webkit do exist; see ‘Epiphany’, ‘surf’, ‘luakit’, and ‘Nyxt’. Qt’s web component used to be based on webkit as well, though they’ve switched to Blink (Chromium).
Unfortunately, none of the browsers listed above are 100% sufficient to replace Firefox. They all rely on GTK bindings on webkit, which has its own quirks; and none have support for webextensions.
Who’s “we”, though? Here’s the list of Linux Foundation members: https://www.linuxfoundation.org/about/members It’s a foundation by, and for, commercial interests; not the users. If the same interests made up a foundation to develop a browser, it wouldn’t be different from Chrome; because in the realm where browsers are supposed to work, those ‘commercial interests’ would demand doing what Chrome does.
It’s a ‘happy accident’ that with respect to a unix-like OS kernel, the interests of the industry ended up being compatible with the interests of the user.
Santagate 2019 Pro for Workgroups
Wouldn’t enabling the --system-site-packages
flag during venv creation do exactly what the OP wants, provided that gunicorn is installed as a system package (e.g. with the distro’s package manager)? https://docs.python.org/3/library/venv.html
Sharing packages between venvs would be a dirty trick indeed; though sharing with system-site-packages
should be fine, AFAIK.
Michael W. Lucas’s “Networking for System Administrators” is a great resource: https://mwl.io/nonfiction/networking#n4sa
PyMuPDF is excellent for extracting ‘structured’ text from a pdf page — though I believe ‘pulling out relevant information’ will still be a manual task, UNLESS the text you’re working with allows parsing into meaningful units.
That’s because ‘textual’ content in a pdf is nothing other than a bunch of instructions to draw glyphs inside a rect that represents a page; utilities that come with mupdf or poppler arrange those glyphs (not always perfectly) into ‘blocks’, ‘lines’, and ‘words’ based solely on whitespace separation; the programmer who uses those utilities in an end-user facing application then has to figure out how to create the illusion (so to speak) that the user is selecting/copying/searching for paragraphs, sentences, and so on, in proper reading order.
PyMuPDF comes with a rich collection of convenience functions to make all that less painful; like dehyphenation, eliminating superfluous whitespace, etc. but still, need some further processing to pick out humanly relevant info.
Built-in regex capabilities of Python can suffice for that parsing; but if not, you might want to look into NLTK tools, which apply sophisticated methods to tokenize words & sentences.
EDIT: I really should’ve mentioned some proper full text search tools. Once you have a good plaintext representation of a pdf page, you might want to feed that representation into tools like the following to index them properly for relevant info:
https://lunr.readthedocs.io/en/latest/ – this is easy to use, & set up, esp. in a python project.
… it’s based on principles that are put to use in this full-scale, ‘industrial strength’ full text search engine: https://solr.apache.org/ – it’s a bit of a pain to set up; but python can interface with it through any http client. Once you set up some kind of mapping between search tokens/keywords/tags, the plaintext page, & the actual pdf, you can get from a phrase search, for example, to a bunch of vector graphics (i.e. the pdf) relatively painlessly.
Another vote for Tesseract – just to clarify the terminology, though: PDF is a fragile format best used read-only; so you really don’t want to edit a pdf, but make a new one using the same (or cleaned-up) bitmaps and a new ocr text layer.
Now, tesseract is excellent at recognizing glyphs; but especially if the scanned image is a little fuzzy, the layout detection falters; and when it falters, you get redundant line breaks, & chunks of text in the wrong order – all of which gets incredibly annoying for searching & copying purposes. So if you can spare the time, and the text requires it, you may need to mark regions (paragraphs & titles mainly) on the bitmap image manually. There exist a few frontends to Tesseract that help with a task like that; check out, e.g., https://github.com/manisandro/gImageReader - inside single paragraph blocks of text, Tesseract doesn’t get as easily confused; and the text output is in the correct reading order, & w/o redundant breaks.
Recently I became aware of ‘StarLite’ tablets – the prices are pretty steep, but the specs look really good, esp. wrt the screen.
Though ‘finding’ the UDP packet should cost a lot more, because, whoever knows where it is?